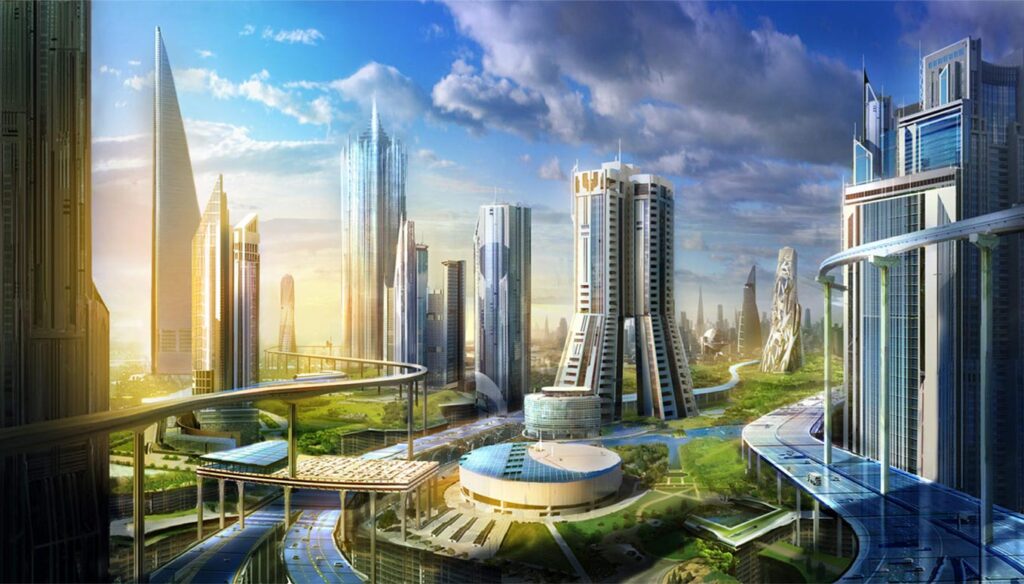
As urban populations continue to grow, cities around the world face the challenge of managing increasingly complex infrastructure, transportation systems, and public services. The rise of smart cities aims to address these challenges by leveraging technology and data to optimize urban living. At the heart of this transformation lies spatial data management—an essential component in the smart city framework that enables efficient urban planning, real-time monitoring, and data-driven decision-making.
The Role of Spatial Data in Smart Cities
Spatial data, which includes geographic information about the location and characteristics of natural or man-made features, is fundamental to the functioning of smart cities. It powers various applications, from traffic management and emergency response to environmental monitoring and public service delivery.
- Urban Planning and Development: Spatial data allows city planners to visualize and analyze the geographic distribution of resources, infrastructure, and population density. This information is critical for making informed decisions about land use, zoning, and the placement of new infrastructure projects such as roads, schools, and healthcare facilities. Advanced Geographic Information Systems (GIS) enable the simulation of various urban development scenarios, helping cities anticipate future needs and avoid potential issues.
- Transportation and Mobility: Efficient transportation is a cornerstone of smart cities. Spatial data management systems provide real-time data on traffic flow, public transportation usage, and pedestrian movement. This information can be used to optimize traffic signals, manage congestion, and design more efficient public transportation routes. Additionally, spatial data is vital for the development and deployment of autonomous vehicles, which rely on accurate and up-to-date maps to navigate urban environments.
- Public Safety and Emergency Response: In emergencies, every second counts. Spatial data enables first responders to quickly locate incidents, assess the situation, and determine the best routes to the scene. For instance, during natural disasters, spatial data can help map out affected areas, identify evacuation routes, and coordinate relief efforts. Moreover, by integrating spatial data with predictive analytics, cities can identify high-risk areas and implement preventive measures to enhance public safety.
- Environmental Monitoring and Sustainability: Smart cities aim to create sustainable urban environments. Spatial data is essential for monitoring air and water quality, tracking energy consumption, and managing waste. For example, spatial data can help identify areas with high levels of pollution and direct efforts to mitigate environmental impact. Furthermore, spatial data supports the development of green infrastructure, such as parks and renewable energy installations, by identifying suitable locations based on environmental and social factors.
Challenges in Spatial Data Management
Despite its importance, managing spatial data in smart cities comes with challenges.
- Data Integration: One of the primary challenges is integrating data from various sources. Smart cities rely on a vast array of sensors, devices, and databases that generate spatial data in different formats. Ensuring that these data sources are compatible and can be seamlessly integrated into a unified system is crucial for effective data management.
- Data Privacy and Security: As smart cities collect and analyze vast amounts of data, ensuring the privacy and security of citizens’ information is paramount. Spatial data often includes sensitive information, such as the location of individuals or critical infrastructure. Implementing robust data protection measures and adhering to privacy regulations are essential to maintaining public trust.
- Scalability: The volume of spatial data generated in a smart city is immense, and it continues to grow as more devices and sensors are deployed. Managing this data efficiently requires scalable storage and processing solutions. Cloud computing and edge computing are increasingly being used to handle the scale of spatial data in smart cities.
The Future of Spatial Data in Smart Cities
As technology evolves, the capabilities of spatial data management will continue to expand. The integration of artificial intelligence (AI) and machine learning (ML) with spatial data will enable more sophisticated analysis and predictive modeling. For example, AI could be used to predict traffic patterns based on historical data and real-time inputs, allowing cities to proactively manage congestion.
Furthermore, the development of 5G networks will enhance the ability of smart cities to collect and process spatial data in real-time, supporting applications such as autonomous vehicles, drone deliveries, and smart grid management.
Spatial data management is a critical component of the smart city framework, enabling cities to navigate the complexities of urbanization effectively. By harnessing the power of spatial data, cities can optimize their infrastructure, improve public services, enhance sustainability, and ultimately create a better quality of life for their residents. However, addressing the challenges of data integration, privacy, security, and scalability will be key to realizing the full potential of spatial data in the cities of the future.